Artificial intelligence and machine learning are groundbreaking technologies reshaping the world around us, paving the way for a fully digital society and the information era. And it doesn’t stop there. A and big data analytics have now enabled us to handle and process terabytes of torrential data generated across myriad scientific and technological domains.
Want to learn how AI has begun to play a more central role in scientific research and development? Well, then, do read this blog. It comes straight from the post-grad subject matter experts of MyAssignmentHelp.expert, the USA’s largest science assignment help service. It offers a detailed overview of the most prominent applications of AI in scientific R&D.
AI In Information Science
AI attempts to give robots the ability to perceive, think, and make decisions. New research and applications in information science are emerging at an unprecedented rate, which is inextricably linked to the AI infrastructure. An AI infrastructure layer comprises data, storage, processing power, ML algorithms, and the AI framework.
The perception layer allows computers to have fundamental abilities such as vision and hearing. For example, computer vision allows machines to “see” and identify things, whereas voice recognition and synthesis let machines “hear” and distinguish speech parts. The cognitive layer enhances induction, reasoning, and information acquisition through NLP, knowledge graphs, and continuous learning. AI can make optimum judgments at the decision-making layer using techniques such as autonomous planning, expert systems, and decision-supporting systems. Numerous AI applications have significantly influenced basic sciences, industrial production, human life, social governance, and the internet. The following subsections overview the AI framework, automatic machine learning (AutoML) technology, and several cutting-edge AI/ML applications in the information industry.
AI in Networking Research
The use of deep learning in networking has sparked significant attention. Network designers frequently use starting network conditions and/or theoretical assumptions to define real network environments. Traditional network modeling and design, which mathematical models govern, cannot cope with complicated situations, including several flawed and highly dynamic network environments. Integrating DL into network research provides a more accurate description of complicated network settings.
Furthermore, DL might be integrated with the Markov decision process to form the deep reinforcement learning (DRL) model, which determines the best policy based on the reward function and system variables. Combined, these strategies might be used to make better judgments that lead to good network design, ultimately enhancing network quality of service and experience. Regarding the many layers of the network protocol stack, DL/DRL may be used for network feature extraction, decision-making, and so on. In the physical layer, DL is useful for interference alignment. It may also identify modulation modes, create efficient network and error correction codes, etc.
Furthermore, DL might be integrated with the Markov decision process to form the deep reinforcement learning (DRL) model, which determines the best policy based on the reward function and system variables. Combined, these strategies might be used to make better judgments that lead to good network design, ultimately enhancing network quality of service and experience. Regarding the many layers of the network protocol stack, DL/DRL may be used for network feature extraction, decision-making, and so on. In the physical layer, DL is useful for interference alignment. It may also identify modulation modes, create efficient network and error correction codes, etc.
AI in Medical Research
Doctor Watson, built by IBM’s Watson platform, and Modernizing Medicine, which aims to tackle cancer, have now been accepted by CVS and Walgreens in the United States and different medical organizations in China. Doctor Watson uses the IBM Watson platform’s NLP capabilities, which have previously collected massive amounts of medical history and prior knowledge from the literature for reference. After entering the patient’s information, Doctor Watson searches the medical history reserve and generates a basic treatment plan, which is then graded by past knowledge reserves. Doctor Watson makes the final proposal and expresses confidence after storing various models. However, such AI physicians have challenges since their proposals, which rely on existing experience from US institutions, may not be appropriate for other locations with various medical insurance regulations. Furthermore, the Watson platform’s knowledge updating relies heavily on updating the knowledge reserve, which requires manual effort.
Those were some of the biggest applications of AI in the world of scientific research and development. Hope this was an interesting read for everyone. If you are working on an assignment on AI and science and need urgent expert academic writing help, connect with MyAssignmentHelp.expert now!
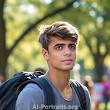