Introduction:
Teaching a machine to listen represents a profound shift in how humans interact with technology. Traditionally, computers processed text-based commands or structured inputs, but enabling machines to interpret and understand spoken language opens a world of new possibilities. This ability allows AI systems to engage in natural conversations, respond to voice commands, and analyze vast amounts of audio data in ways that were previously unimaginable.
The process of teaching machines to listen is rooted in advanced fields like speech recognition, natural language processing, and machine learning. It involves complex algorithms that convert sound waves into meaningful information the machine can act upon. As these technologies mature, the impact spans from everyday conveniences like voice assistants to revolutionary changes in accessibility, healthcare, and customer service.
Understanding the Basics of Machine Listening:
Machine listening involves converting audio input into data that machines can process and understand. At its core, this begins with capturing sound waves through microphones and transforming them into digital signals. These signals are then analyzed using pattern recognition techniques to identify phonemes, words, and ultimately meaning.
The complexity of human speech, including accents, intonations, and background noise, presents significant challenges. Overcoming these requires sophisticated models trained on diverse datasets to recognize speech accurately across different environments and speakers. Machine listening combines acoustics, linguistics, and AI to bridge the gap between raw sound and comprehension.
Exploring the Technologies That Enable Machines to Listen:
Several interrelated technologies work together to teach machines how to listen effectively. Key components include Automatic Speech Recognition (ASR), Natural Language Understanding (NLU), and signal processing techniques that clean and interpret audio input.
- Automatic Speech Recognition (ASR): Converts spoken language into text by identifying phonetic patterns.
- Natural Language Understanding (NLU): Interprets the text to grasp context, intent, and meaning.
- Signal Processing: Enhances audio quality by reducing noise and isolating speech from background sounds.
These technologies rely on machine learning algorithms and neural networks, which learn from vast amounts of audio data to improve accuracy. Continuous advancements in these fields have made voice-activated AI more reliable and widely adopted.
Analyzing the Challenges in Teaching Machines to Listen:
Teaching machines to listen accurately involves overcoming numerous challenges related to variability in speech and environment. Differences in accents, dialects, speech speed, and pronunciation complicate the recognition process. Furthermore, background noise, overlapping voices, and poor audio quality can degrade performance.
Other challenges include understanding context and emotion, which are crucial for truly natural interactions. Machines must not only transcribe words but also infer meaning, detect sarcasm, or recognize sentiment. These subtleties require sophisticated models and ongoing refinement to approximate human-like listening capabilities. As voice-driven technologies become more embedded in customer service, sales, and communication, overcoming these hurdles becomes essential—not just for innovation, but also as a driver of business growth in an increasingly voice-enabled marketplace.
Investigating the Impact of Machine Listening on Human Communication:
Machine listening transforms how humans communicate with technology by enabling voice-based interactions. This shift allows for more natural, hands-free, and accessible interfaces, making technology easier to use for diverse populations, including those with disabilities.
Voice assistants like Siri, Alexa, and Google Assistant exemplify this impact, providing convenience in homes and workplaces. Additionally, machine listening enhances customer service through automated call centers and enables real-time transcription and translation services, breaking down language barriers and improving global communication.
Understanding the Role of Data in Training Listening Machines:
Data plays a pivotal role in teaching machines to listen. Training AI models requires large and diverse audio datasets containing various languages, accents, speaking styles, and environmental conditions. The quality and representativeness of data directly affect the system’s ability to generalize and perform well in real-world scenarios.
Data annotation is equally important, involving the manual labeling of audio clips with transcripts, speaker identity, or emotional tone. This labor-intensive process ensures that models learn to recognize speech accurately and understand contextual nuances. Without rich, well-labeled data, machine listening systems cannot achieve high levels of performance. As these systems evolve, integrating elements like energetic clarity—the ability to detect not just what is said but how it is said—may become essential in applications where emotional resonance, wellness insights, or subtle tone detection are key to deeper, more holistic interactions.
Exploring Ethical Considerations in Machine Listening Technologies:
The ability of machines to listen raises significant ethical questions surrounding privacy, consent, and data security. Voice data is inherently personal and sensitive, often revealing identity, location, and private conversations. Misuse or unauthorized access to such data poses risks to individuals’ privacy rights.
Furthermore, biases in training data can lead to unequal performance across different demographic groups, exacerbating fairness issues. Transparent policies, responsible data handling, and inclusive datasets are essential to address these concerns and build trust in machine listening technologies.
Exploring Practical Applications of Machine Listening Across Industries:
Machine listening is revolutionizing many sectors by enabling new applications and improving existing processes. In healthcare, it supports remote patient monitoring and diagnostic assistance through voice analysis. In customer service, automated voice bots handle inquiries efficiently, reducing wait times.
Other notable applications include:
- Education: Providing personalized tutoring and accessibility for students with disabilities.
- Automotive: Enabling voice-controlled navigation and hands-free communication.
- Security: Monitoring environments for unusual sounds or threats.
These diverse use cases demonstrate the broad potential of machines that can listen and respond intelligently. Many organizations rely on robust cloud platforms to deploy and maintain their machine listening solutions efficiently. Leveraging professional azure support services ensures reliable performance, scalability, and seamless integration of AI technologies within existing infrastructures.
Learning How to Build Skills in Machine Listening Through Structured Courses:
Developing expertise in machine listening requires a strong foundation in AI, signal processing, and linguistics. Participating in specialized training, can equip learners with essential skills to design effective AI-human interactions. These courses often cover core concepts, practical tools, and hands-on projects that deepen understanding.
For those interested in advancing their knowledge, enrolling in a prompt engineering course offers a structured pathway to master the art of crafting instructions that optimize AI listening and response capabilities. This education is crucial in an era where voice-driven technologies are rapidly expanding.
Understanding the Importance of Multilingual Capabilities in Machine Listening:
As global communication grows increasingly interconnected, the ability for machines to listen and understand multiple languages becomes crucial. Multilingual machine listening enables AI systems to serve diverse populations, breaking down language barriers and facilitating smoother interactions. This capability requires training models on extensive datasets encompassing various languages, dialects, and cultural nuances.
Achieving effective multilingual listening presents unique challenges, such as handling code-switching (when speakers alternate between languages) and adapting to regional accents. Nonetheless, it greatly expands the accessibility and usability of voice-activated technologies worldwide, fostering inclusivity and broadening AI’s reach.
Exploring How Machine Listening Enhances Accessibility for People with Disabilities:
Machine listening technologies play a vital role in improving accessibility for individuals with disabilities. Voice-controlled interfaces allow users with mobility impairments to interact with devices hands-free, promoting independence. Speech recognition combined with real-time transcription services supports those with hearing impairments by converting spoken language into readable text.
Additionally, AI systems that can interpret vocal cues and emotions help tailor responses to users’ needs, creating more personalized and effective communication aids. By enhancing accessibility, machine listening contributes to a more inclusive digital environment that empowers all users.
Examining Future Trends and Innovations in Machine Listening Technologies:
The field of machine listening is rapidly evolving, driven by advances in AI, computing power, and data availability. Emerging trends include improved contextual understanding, emotional recognition, and integration with other sensory data such as vision and gesture recognition. These innovations promise more sophisticated and human-like listening experiences.
Future developments may also involve decentralized and privacy-focused architectures that protect user data while maintaining performance. As research continues, machine listening will become more adaptive, intuitive, and seamlessly integrated into everyday technologies, shaping the next generation of human-machine communication.
Conclusion:
Teaching machines to listen is a transformative technological advancement that reshapes human-computer interaction. By converting sound into meaningful data, AI systems become capable of natural, intuitive communication, unlocking new possibilities across industries and daily life. Although challenges remain in achieving human-like listening proficiency, ongoing research and innovation continue to push the boundaries.
As voice interfaces and audio-based AI applications proliferate, understanding the mechanics and implications of machine listening becomes essential. Embracing this technology responsibly offers tremendous benefits in accessibility, efficiency, and connectivity, heralding a future where machines truly listen and understand.
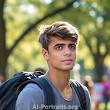